Model, estimate or measure? What matters in video energy use
Analyzing the difference between energy and power estimates from software models vs a hardware analyzer in video communications.
The politicization of climate change has clouded sustainable computing research, often leading to exaggerated claims about technology’s environmental impact. We can see this from the mostly worthless body of literature (over)estimating data center energy consumption1, most recently with an AI-focus.
This distracts from real challenges sourcing enough clean energy, optimizing data center water consumption with the appropriate location context, and dealing with the materials challenges in equipment manufacturing, and recycling.
Amid this noise, I was pleased to read the online publication of Herglotz et al. (2025) on measuring power and energy consumption in video communication. It offers a refreshing, rigorous approach with some interesting conclusions around the appropriateness of using software modeling vs hardware power analyzer devices in different scenarios.
This post discusses how studies like Herglotz et al. (2025) can refocus the field.
Challenges with measuring video energy consumption
Video streaming, a massive driver of network traffic, involves complex systems with multiple hardware and software components. This complexity makes accurate energy measurement challenging, as errors compound across the end-to-end system. This has made it an interesting subject not just for research, but for scrutiny of the environmental impact. The involvement of the natural enemies of the mainstream media, Big Tech, has also made it the perfect target for attack.
The sophistication of these systems is something I spent a lot of time describing in my 2024 paper describing how to model the power consumption of networks, but even that used a simplified example. For real systems like video streaming, there are multiple components making up not just the network hardware but the software components within a data center region.
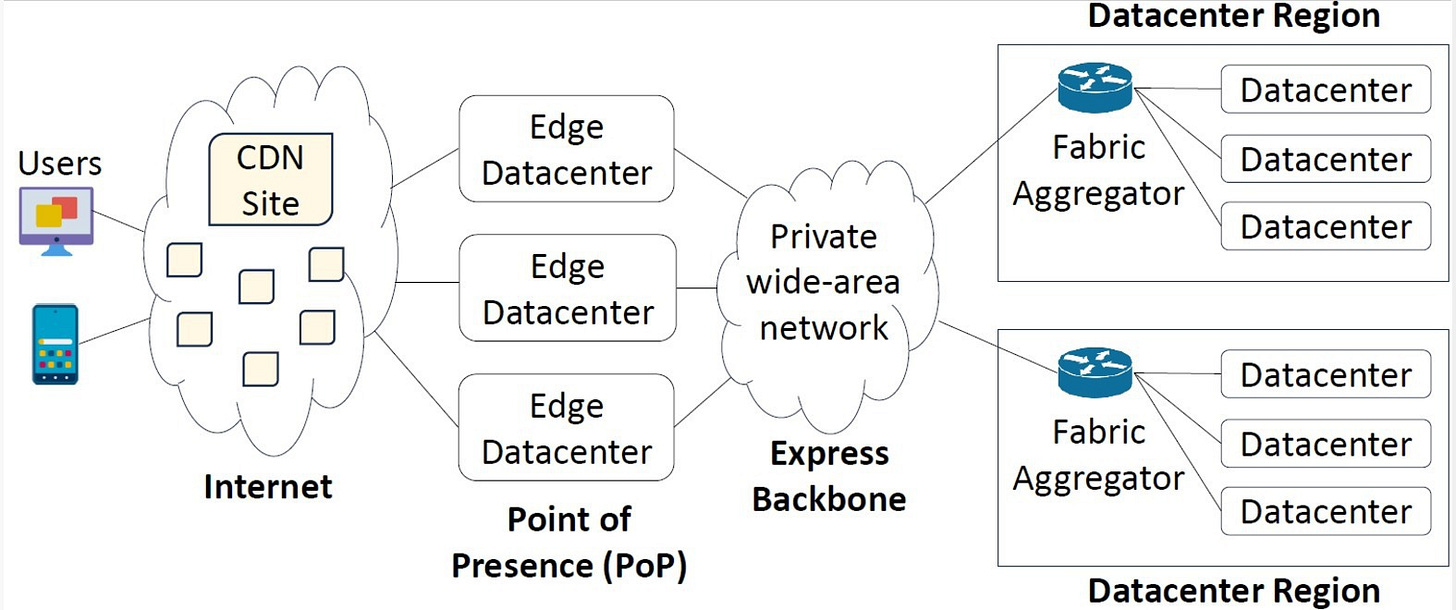
This recent, detailed description of Meta’s infrastructure further strengthens the point I repeatedly make about how energy intensity (kWh/GB) only being a useful metric when looking backwards at known total power consumption and data volumes - it cannot be used to make predictions or estimate the current power consumption of a system.
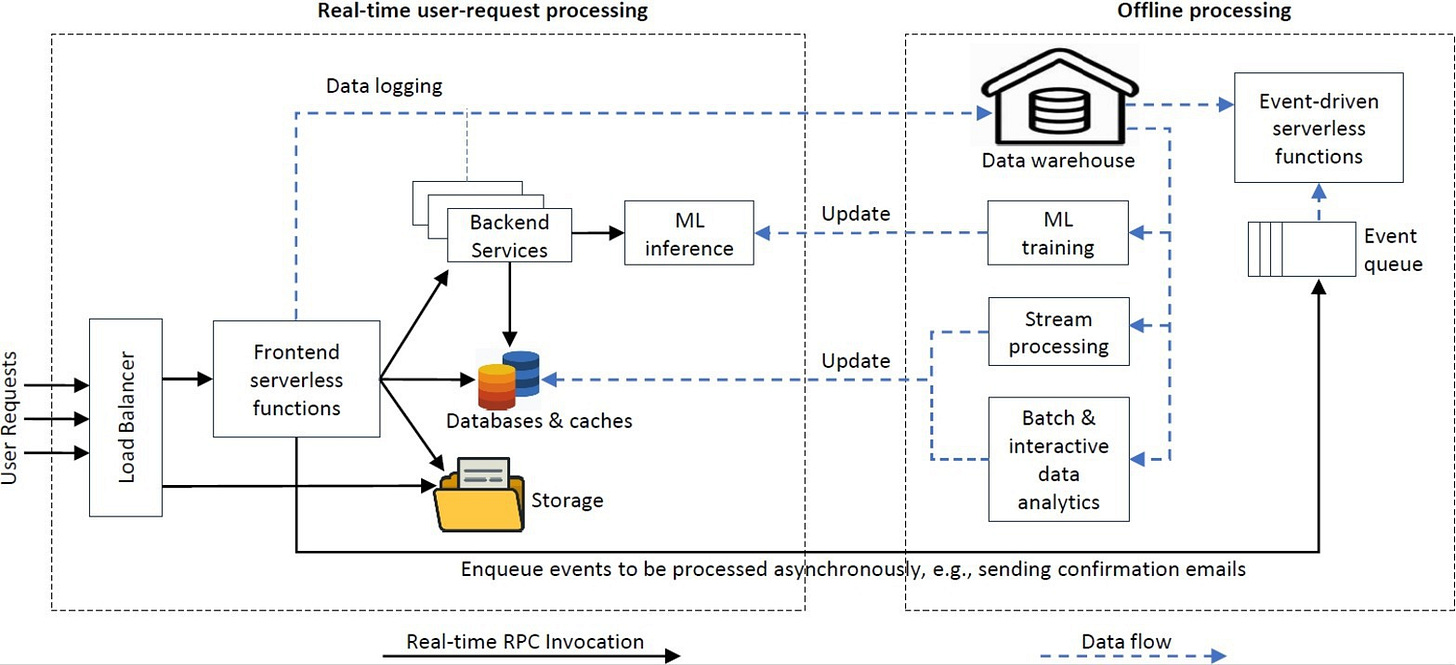
All this is to say that energy estimates of complex end to end systems are highly likely to contain serious errors which compound, something I saw in my review of data center energy estimates between 2007 and 2021.
Measure for absolute accuracy; model for relative comparisons
Herglotz et al. analyze video decoding energy use, comparing software-based RAPL estimates with direct measurements from a Tektronix power analyzer. RAPL, a model built into Intel processors which I have written about previously, estimates energy based on activity counters but lacks transparency and precision.
Real measurements, like those from the power analyzer, offer absolute accuracy—something rare in this field. This distinction matters: models like RAPL are fine for optimization comparisons, but only measurements reveal true energy use.
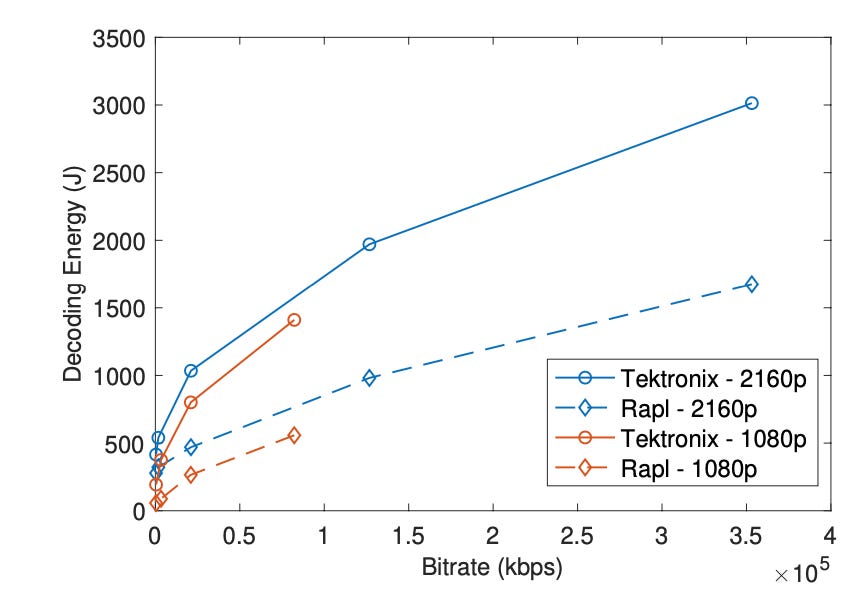
The paper notes that software modeling, like RAPL, is useful when making comparisons for optimization, but contains inherent limitations if used for producing absolute calculations. This is the distinction between measurement and estimation.
Not at all surprising when you think about it, but few publications use real measurements! It’s rare to see this level of detail, including full analysis of the statistical methods that can be used for discussing uncertainty. Again, something I rarely see when reading publications that purport to provide real numbers behind specific activities.
Conclusions
Herglotz et al. stand out for their scientific rigor - offering a concise literature review, a well-defined focus on video decoding, and a methodology that avoids hype. Their use of real measurements and statistical analysis provides a model for tackling complex systems, countering the overestimations that plague the field.
This approach should guide future research, ensuring sustainable computing studies prioritize accuracy over sensationalism. It’s no surprise that some of the authors were also involved in the 2021 Carbon Trust report on the carbon impact of video streaming, which I consider to be the definitive analysis.
We long ago reached the point where software systems such as video streaming evolved past their ability to be modelled without detailed inside information. The academic literature has still not fully caught up - there are always opportunities for better transparency - but Herglotz et al. shows how to analyze specific components of those systems.
There are only 2 credible research groups involved in data center energy estimates: those represented by Masanet, Shehabi, et al (through Berkeley Lab), and Borderstep. See my recent writeup of data center energy in 2025.